From Adaptive to Adaptable: The Next Generation for Personalized Learning
This paper comprises several sequential articles contributed by participants in the 1EdTech Consortium Adaptive Learning Innovation Leadership Network.
Melissa Edwards, Purdue University | Dale Johnson, Arizona State University |
Cristi Ford, University of Maryland, University College | Lou Pugliese, Arizona State University |
John Fritz, University of Maryland, Baltimore County | Samantha Birk, 1EdTech Consortium |
Important Links |
---|
Table of Contents
Introduction: A Personal Example of Why Adaptive Learning Works
Introduction: A Personal Example of Why Adaptive Learning Works
When I first started my Ph.D. eight years ago, I had to make an early decision that I knew would loom large: was my dissertation going to be a qualitative or quantitative study? Having studied English in college and grad school, a qualitative study might have better suited my skill set, but since I was interested in doing a data mining study of our campus’ learning management system (LMS), a quantitative study made more sense methodologically. My only concern was a spotty math background due in part to a cross-country move as a teenager and attending three different high schools. I knew I could write, but my preferred dissertation topic meant I also had to compute. And that meant I had to fill some gaps I’d simply learned to work around for too long.
Fortunately, I was blessed with an encouraging, patient professor for my two doctoral stats classes. I was okay with key concepts and could follow her procedural steps in class, but I struggled to replicate them on my own or on exams. About this time I came across Sal Khan’s fascinating 2011 TED talk on how he built Khan Academy (KA) based on lessons learned from tutoring his cousin with short screencasts of him working out problems that he put on YouTube1. Like his cousin and many others since, I loved watching Sal explain concepts I was fuzzy about, like linear regression, interaction effects, or the Central Limit Theorem. Also, I could play, pause and replay him over and over again, on my own time. Better yet, by the time I stumbled across KA, he had developed practice problems I could use to apply the concepts he explained. If I answered ten in a row correctly, I was declared “proficient” and encouraged to move on to a new topic. If I got stuck, I could watch a related video that would only pause my 10-in-a-row streak, or request a hint that made me start a new one. I also enjoyed his The One World School House (2012) and cited it in my dissertation that I completed in 2016.
Why is this important?
At its best, adaptive learning is really just a means to an end: developing a student’s ability to honestly and accurately self-assess his or her current knowledge, skills or abilities. Indeed, Barry Zimmerman has described two keys to students becoming self-regulated learners: (1) a willingness to take ownership of his or her conceptual problems, and (2) remediation that is focused and specific to the student’s particular gap, instead of general aphorisms such as “study harder."2 Adaptive learning is really about the remediation environment we provide to our students through course design and related technology (#2), but it can’t work unless students are “ready” (#1). Like others who have extolled the virtues of Khan Academy,3 I was ready and able to use it for my Ph.D. data mining study because I knew I had deficits. But it only worked because it could tailor itself to help address specific gaps I demonstrated in my answers to its practice questions.
While I’m a KA fan, adaptive or personalized learning or even “programmed” or “differentiated” instruction has been around ever since B.F. Skinner’s teaching machine.4 I even remember a programmed instruction grammar textbook I used in Freshmen English, and some may enjoy Audrey Watters’ short history of the Science Research Associates (SRA) “reading boxes” that were popular in grade school classrooms during the sixties and seventies.5 If you’ve never used Khan Academy, of course, I recommend it, but even more so for this white paper about adaptive learning. Assuming the old adage is true—that “teachers teach the way they were taught”—I’m going to also assume that most weren’t taught with adaptive learning. If so, Khan Academy is a great way to experience what adaptive learning is like as a student, to help think about how to use adaptive learning as an instructor, or someone who supports one.6
And yet, not everyone is a fan of Khan Academy, including some instructors who question its accuracy.7 My daughter’s high school math teacher even said she should be careful because it is “too professional,” whatever that means. Perhaps more importantly, Khan Academy has not always been easy to integrate into other campus systems likely to be in use by instructors. All this is to say that like any pedagogical innovation, we need to understand what it is and an inevitable “build vs. buy” decision we must make if we want to scale. The deep dives that follow by Dale Johnson and Lou Pugliese from Arizona State University should really help institutions frame the pros and cons of this question.
In my opinion, adaptive learning is the holy grail of educational technology—well, besides a Holodeck, of course. Instead of one-size fits all approaches to teaching and learning, adaptive learning can personalize learning at scale. We’re still in the early stages, but adaptive technologies can help facilitate responsibility for learning, which is ultimately what distinguishes a student from a customer.
______________________________
1 https://youtu.be/gM95HHI4gLk
2 http://chronicle.com/article/Struggling-Students-Can/64004/
3 https://www.khanacademy.org/stories
4 http://americanhistory.si.edu/collections/search/object/nmah_690062
5 http://hackeducation.com/2015/03/19/sra
6 You can pick any subject, but I suggest the KA “class” on probability. Start by selecting “test yourself.”
7 http://www.chronicle.com/blognetwork/castingoutnines/2013/10/23/khan-academy-using-contractors-for-accuracy-checking-some-thoughts/
Making a Case for an Adaptable Learning Platform
By Dale Johnson, Adaptive Program Manager, Arizona State UniversityThe growing use of adaptive courseware has led to significant questions about the role of faculty in the process of developing, validating, and using them in their courses. Statements about artificial intelligence and advanced algorithms replacing instructors increase the risk of this discussion devolving into an unproductive “people versus technology” debate. Early efforts to create and implement adaptive courseware have clearly demonstrated that faculty are critical to the process and that these systems function best as part of an educational ecosystem—not as separate entities for educating students.
Faculty have always been the principal creators and disseminators of the knowledge at the heart of the educational process. By necessity, they play the same role in the development of adaptive courseware. Written explanations of complex concepts, videos to elucidate ideas, and assessment activities to challenge students are all examples of components in adaptive systems being generated by the creative minds of the faculty. They are the ones responsible for organizing this content into coherent lessons and sequencing those lessons for effective educational outcomes, whether that is in a traditional course or in an adaptive courseware.
To increase the adoption of adaptive courseware, we will need to answer the question, “what is the most effective way to help faculty use adaptive systems that can improve educational outcomes?” It is physically and financially unrealistic for each instructor to create a unique adaptive system. That would be equivalent to each one writing their own textbook. However, it is realistic to believe that each may contribute their knowledge to the creation of a better courseware for their students. Scholar-sourcing information from thousands of faculty members could provide a new model for developing these next generation, “adaptable” educational systems.
Early in my work on adaptive courseware, I had an experience that highlighted the need for adaptable systems that faculty can work with in order to create adaptive courseware for the students.
The lead faculty member on an introduction to biology course was a professor who had taught this subject to over 30,000 students during his career. Based on his experience, he said that students learn this subject better when the concepts are ordered from “macro” (biomes) to “micro” (DNA) in the curriculum. We videotaped all of his lectures in that sequence, and the instructional design team configured the courseware as directed. After two semesters in that format, a new lead faculty member was assigned to the course. She had different ideas about the best way to teach introduction to biology and proceeded to reconfigure the lesson sequence from micro to macro. Making those changes in our courseware consumed most of our four-week winter break. We had to move every piece of content and assessment to its new location through a very tedious manual process and then rewrite all of the exams to align with the new curriculum. That inefficient process opened our eyes to the need for more nimble systems that can be adapted by faculty members as they learn what works best for the students.
That is why a primary objective of the 1EdTech adaptive courseware Innovation Leadership Network is to create adaptable systems that can be reconfigured easily by the faculty as they experiment and learn what works for students. The design of that system has to start at the core with a standard definition of a “lesson” which can be moved in a system or shared among systems. For our purposes, a lesson is defined as a single learning objective supported by multiple instructional resources and assessment activities.
An adaptable system will allow the faculty to configure these lessons into a curriculum and test the efficacy of each of the components as well as the whole. It will support the scientific process of hypothesizing, creating, testing, and improving the educational outcomes from the system.
The Visualization for an Ideal Adaptable Learning Ecosystem
By Lou Pugliese, Director, Action Lab, Arizona State UniversityAdaptive learning is a technology and data-driven system of integrating instructional resources, learning objectives, and assessment activities into single, progressive modular learning elements that can be adapted to individual learners, reordered, or shared between learning systems. In short, the adaptive learning platform market is in the nascent stage of development, which creates confusion about what “adaptive” or “personalized” learning really is, what vendors are developing to what purposeful use and why faculty should be compelled to engage in experimentation. This environment of uncertainty causes apprehension among early adopter faculty impeding the advancement and benefits of adaptive learning into the mainstream of higher education. While there are broad applications for adaptive architecture for varying content, students and course objectives, we have attempted to define the following four general adaptive categorical frameworks representing various levels of complexity;
Questions and Problems We are Trying to Solve to Reach an Adaptive Learning Ecosystem
By the 1EdTech Consortium Adaptive Learning Innovation Leadership NetworkThe diverse categorical frameworks offered in adaptive learning make evident that there is a need for a collaborative of key stakeholders to express the next iteration of requirements necessary in this adaptive learning space. The Adaptive Learning Innovation Leadership Network has established the following set of criteria and questions for further exploration by the field with the intention of engaging the vendor community on what the faculty can continue to engage in meaningful work in adaptive learning. The following areas offer some insights to the necessary challenges we hope to address and offer future directions of the adaptive learning ecosystem.
- Able to adapt and conform to a non-traditional learner lifestyle in which the pace is variable and the learner has on-demand requirements.
- Have statistically accurate cognitive models representing authenticity of skill and competency mastery.
- How does the system achieve that adaptive state?
- Have accurate content path placement that includes accurate assessment of learned knowledge that can be applied.
- Able to correctly perform adaptive sequencing to precisely and continuously collect real-time data on a student’s performance and use it to automatically change a student’s learning experience.
- Have this data in a sharable format.
- Have the ability to accurately determine remediation and corrective action using adaptive assessments in both normed and criterion-referenced assessment design where applicable.
- Have the correct algorithm design for weighing different multiple measurement factors.
- Can you describe how the adaptive logic works in your system?
- Designed using adaptive assessments or are assessments derived from traditional formative assessment item banks.
- Have synchronous capabilities as a critical measurement of student engagement behavior, and content knowledge mastery.
- Have the necessary provisioning architecture to scale over thousands of concurrent users when considering the complex calculations needed to accommodate adaptive learning.
- Able to develop comprehensive competency frameworks that index learning standards and outcomes.
- Able to articulate the relationship between adaptive learning models and competency-based framework.
- Multiple levels of competencies, based on outcomes, rather than on assessments?
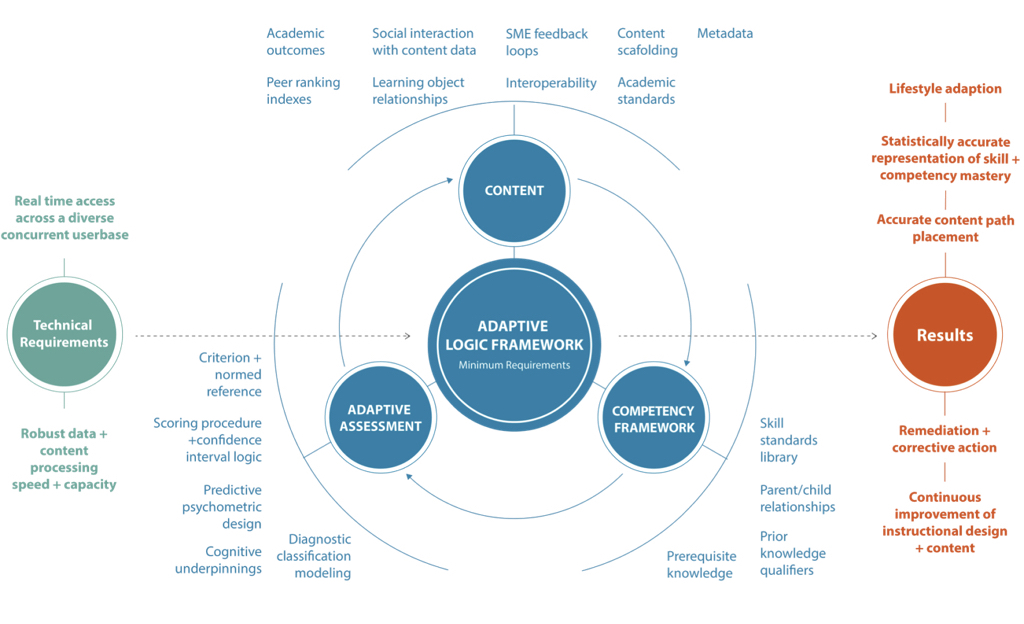
Figure 1. A conceptual model of the ideal adaptive/adaptable learning environment framework developed by Lou Pugliese, Director, Action Lab, Arizona State University
1EdTech Standards for an Adaptive/Adaptable Learning Ecosystem
By Samantha Birk, HED Institutional Program Manager, 1EdTech ConsortiumIn a cohesive, extensible adaptive learning environment the interoperability of multiple technology systems is the linchpin to success. In an ideal configuration, systems, both campus and third-party, work in concert leveraging open interoperability standards to provide a seamless experience for student and instructor, while ubiquitously leveraging multiple data points to inform the learning flow. This conceptualization of the next generation adaptable learning environment has as its foundation a three-layer architecture for organizing instructional resources:
- Concept Chart
- Course Configuration
- Content Curation
It is theorized that leveraging four of the 1EdTech standards when innovatively used in concert with a revisioning of an architecture for an adaptable learning ecosystem, a clear path is laid out.
This shift would be characterized as interoperable, seamlessly allowing institutions to cohesively utilize multiple adaptable systems on campus and empower the faculty with greater pedagogical choices that have the:
- Ability to support flexible course design and content sequencing that can be easily manipulated by the instructor to meet pedagogical requirements and learning outcomes.
- Collect and aggregate multiple data collection points, provide a holistic understanding of a student’s learning style and abilities.
- Remove the limitations that proprietary systems create (walled gardens) that silo assessment, algorithms, and learning data.
- Achieve this ideal immersive adaptable learning environment requires leveraging some open interoperability standards.
Learning Tools Interoperability (LTI)
What it is:
In its basic form, LTI enables the user to move from one system to another without logging in.
Features:
- Creates a seamless experience for the faculty and student to move from the LMS into a third-party tool through a secure pass of use data that informs the tools the user's role (e.g. student), ID, etc.
- Ability to pass grade data from the adaptive system to the LMS.
Current limitations:
- None identified at this time.
Role in Adaptable Learning Ecosystem
How students may access an adaptive or adaptable learning system directly, but more than likely they will be moving from an LMS or similar campus learning system to the adaptive content when the adaptive content is only available in a third-party platform. In this scenario, LTI would be used to seamlessly pass the student from the LMS into the adaptive content.
Figure 2. Basic Adaptable Learning Ecosystem using LTI Integration
Scenario 2:
In many instances, instructors need student grades captured while working in the third-party tool reported back into the LMS, usually to the course gradebook. Leveraging LTI and the Gradebook Extension supports the launch and pass of the student from the LMS into the adaptive or adaptable system while passing grades back from the system into the LMS.
Figure 3. Basic adaptable learning ecosystem using LTI integration and Gradebook Service
Caliper Analytics
What it is:
- A means for consistently capturing and presenting measures of learning activity in a common data format that when analyzed using a set of metric profiles, directs the learning path.
Role in Adaptable Learning Ecosystem
Adaptive and adaptable learning cannot function without the collection and analysis of student learning data. Data and analytics are the linchpins of an adaptive learning ecosystem. All current adaptive systems have been built around proprietary standards and algorithms that reinforce the silos, or "walled gardens." These make it nearly impossible for the educator, student or institution to see a truly holistic view what is happening across the learning environment. Since many curriculums ask students to work in multiple learning environments (and possibly multiple adaptive learning systems), allowing data to be collected, combined with other provider data points, shared and analyzed in a consolidated view is the only true way to understand the student's progress within the curriculum fully. As an open standard, Caliper Analytics supports this aggregation of cross-provider data and enables the institution to see more clearly and deeply into the learning process.
- In the current environment, no data is passed back to the LMS or the institutions regarding the student's progress through the adaptive system. Student(s) scores might be passed back via LTI Gradebook Services, but there's no learning data that the institution can use to track student progress across multiple systems (e.g. how many pages were read, what chapter was not completed, how many simulations were completed, etc.) or other data points that would inform and trigger academic interventions.
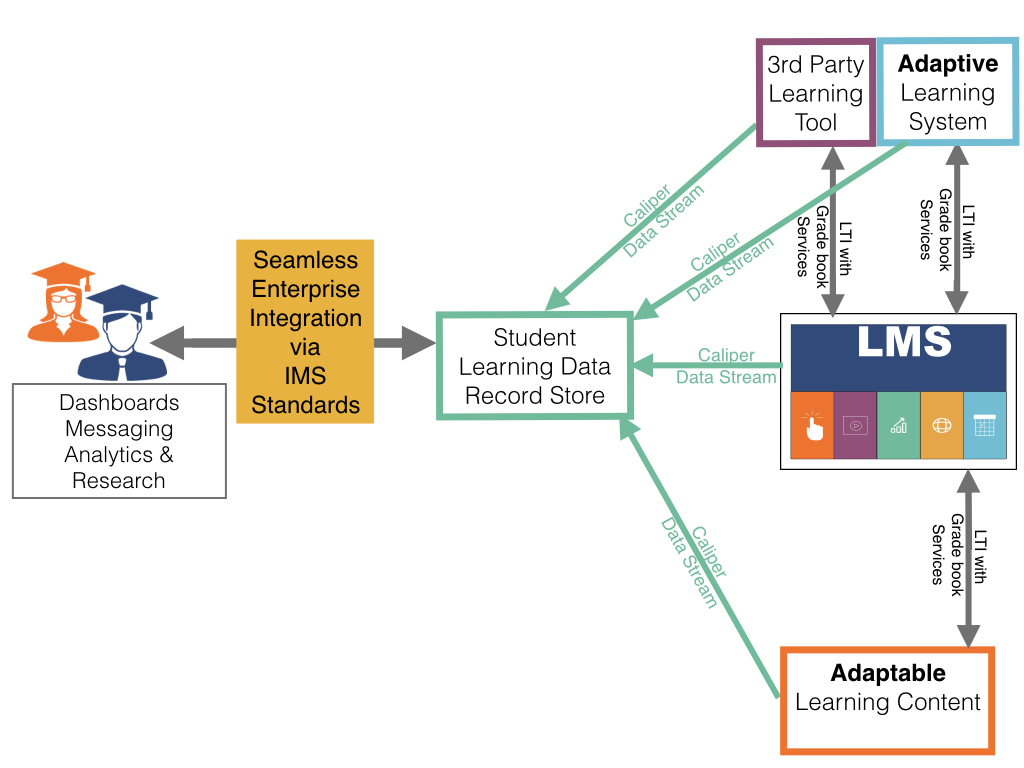
Common Cartridge (CC) and Thin Common Cartridge (Thin CC)
- CC - a standardized way to package, import, export, and exchange digital learning materials and assessments from an LMS, portal, learning object repository or other learning platforms.
- Thin CC - a standardized way to exchange links and provide authorization to third party web-based learning tools via Learning Tools Interoperability.
Role in Adaptable Learning Ecosystem
Common Cartridge and Thin Common Cartridge are related standards and are a way to package and exchange digital learning materials and assessments. Most often, CC is used to import and export course materials to and from your LMS, learning portal, LOR (Learning Object Repository) or another platform. Since course content can include large files or those that need to be maintained on an external server Thin CC is used to exchange links and seamless access to third party web-based learning content via LTI. It is most commonly used to package publisher content and is an easy way to add this content to a course, often saving faculty and instructional designers countless hours of content development time.
- Content cannot be rearranged.

Question and Test Interoperability (QTI) and Accessible Portable Item Protocol (APIP)
- QTI and APIP are two tightly connected standards. QTI enables the interoperability of assessment items and tests and is used in Common Cartridge and formats assessments for importing and exporting content into/out of learning platforms.
Role in Adaptable Learning Ecosystem
APIP uses QTI as the base assessment format and adds support for rich accessibility features that meet the personal needs and presentation preferences of students with a variety of accommodation requirements. QTI v3 (under development now) adds the accessibility functionality introduced in APIP and improves upon it with the latest web accessibility and HTML standards developed by the W3C.The pivot point for adaptive learning is the assessment items which govern the prescriptive next steps for a student in an adaptive learning system. The current limitation is that the algorithms that inform the learning path are tied to prescribed assessments, allowing for minimal if any content changes to support instructor and pedagogical choices. In an adaptable ecosystem, the marriage between algorithm and assessment item would remain intact but would incorporate a level of logic that would enable content to be reordered or subsidized as deemed by the instructor, designer, program manager or other authorized curriculum decision maker.
- Neither the QTI or APIP standards accommodate an affiliation with a learning algorithm needed to prescribe a student’s overall learning path that is adaptable and characterizes an adaptive learning experience. QTI does support testing through Computer Adaptive Testing (CAT). The CAT standard is intended to support learning algorithms that can be connected to a number of systems or data sets such as psychometric data, constraints, stopping criteria, and others. It enables an assessment, such as an exam, to present increasingly more difficult or easier questions based on how the student is performing on the assessment.

Select Terms
Select Additional Resources
- Learning to Adapt 2.0: The Evolution of Adaptive Learning in Higher Education, April 2016, Tyton Partners: http://tytonpartners.com/library/learning-to-adapt-2-0-the-evolution-of-adaptive-learning-in-higher-education/
- Lessons Learned from Early Implementations of Adaptive Courseware, 2016, SRI Education: https://www.sri.com/work/publications/lessons-learned-early-implementations-adaptive-courseware
- Empowering Educators Means Decoding Adaptive, Edsurge (2016), Pearson: https://www.edsurge.com/news/2016-05-10-empowering-educators-means-decoding-adaptive-learning
- “CwIC” framework from Tyton Partners for Gates Foundation (http://coursewareincontext.org)